Cerebras Systems Inc. was founded in 2016 by Andrew Feldman, Jean-Philippe Fricker, Michael James and Sean Lie and is doing business in building computer systems for complex artificial intelligence deep learning applications. As of October 2021, Cerebras Systems has a market valuation of more than $270 million.
Cerebras Systems has a total of 102 patents globally, out of which 34 has been granted. Of these 102 patents, more than 85% patents are active. USA is where Cerebras Systems has filed maximum number of patents, followed by Europe and Japan and it also seems reasonable as the biggest market for Cerebras Systems is the United States, it has generated an annual revenue of $10-$100 million. Parallelly, United States seems to be the main focused R&D center and is also the origin country of Cerebras Systems.
Do read about some of the most popular patents of Cerebras Systems which have been covered by us in this article and also you can find Cerebras system’s patents information, the worldwide patent filing activity and its patent filing trend over the years, and many other stats over Cerebras System’s patent portfolio.
How many patents does Cerebras Systems have?
Cerebras Systems has a total of 102 patents globally. These patents belong to 15 unique patent families. Out of 102 patents, 89 patents are active.
How many Cerebras Systems patents are Alive/Dead?
Worldwide Patents
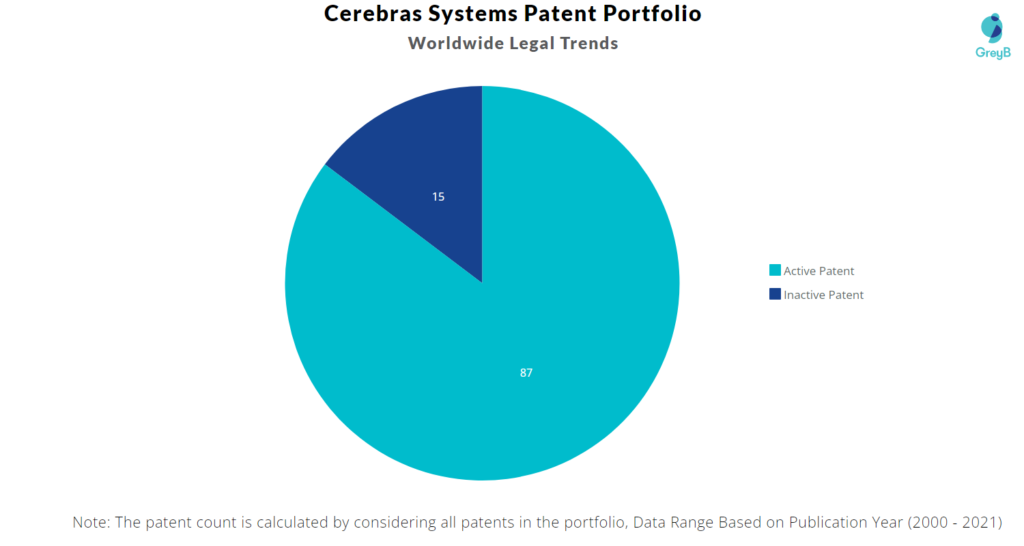
Patent Families
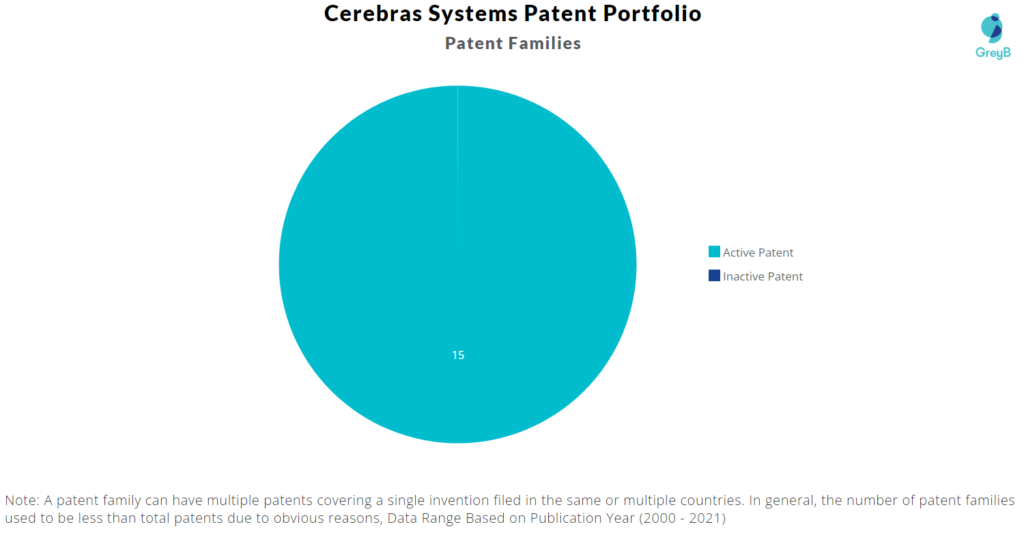
How Many Patents did Cerebras Systems File Every Year?

Are you wondering why there is a drop in patent filing for the last two years? It is because a patent application can take up to 18 months to get published. Certainly, it doesn’t suggest a decrease in the patent filing.
Years of Patent Filing or Grant | Cerebras Systems Application Filed | Cerebras Systems Patents Granted |
2021 | 10 | 14 |
2020 | 21 | 13 |
2019 | 21 | 7 |
2018 | 50 | – |
How Many Patents did Cerebras Systems File in Different Countries?
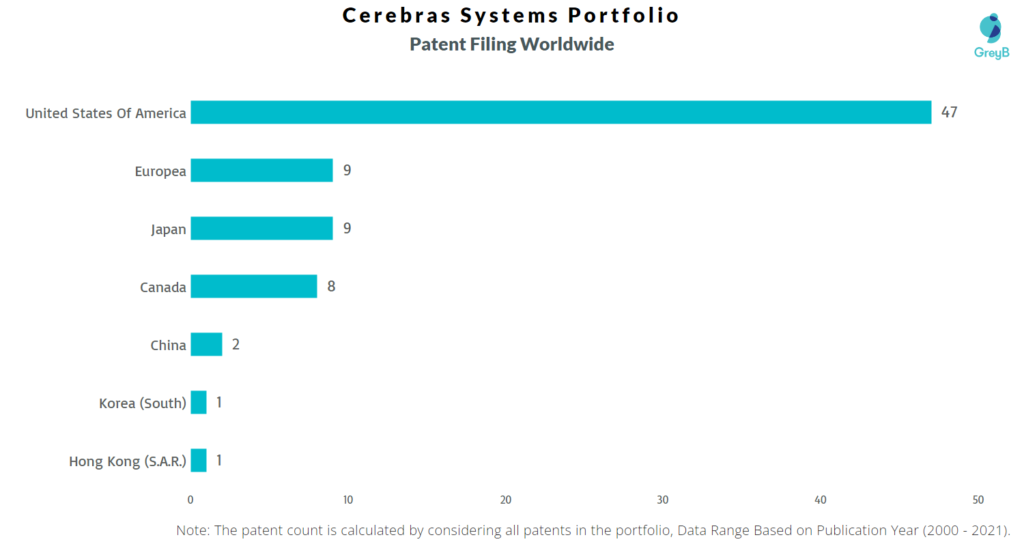
Countries in which Cerebras Systems Filed Patents
Country | Patents |
United States Of America | 47 |
Europe | 9 |
Japan | 9 |
Canada | 8 |
China | 2 |
Korea (South) | 1 |
Hong Kong (S.A.R.) | 1 |
Where are Research Centers of Cerebras Systems Patents Located?
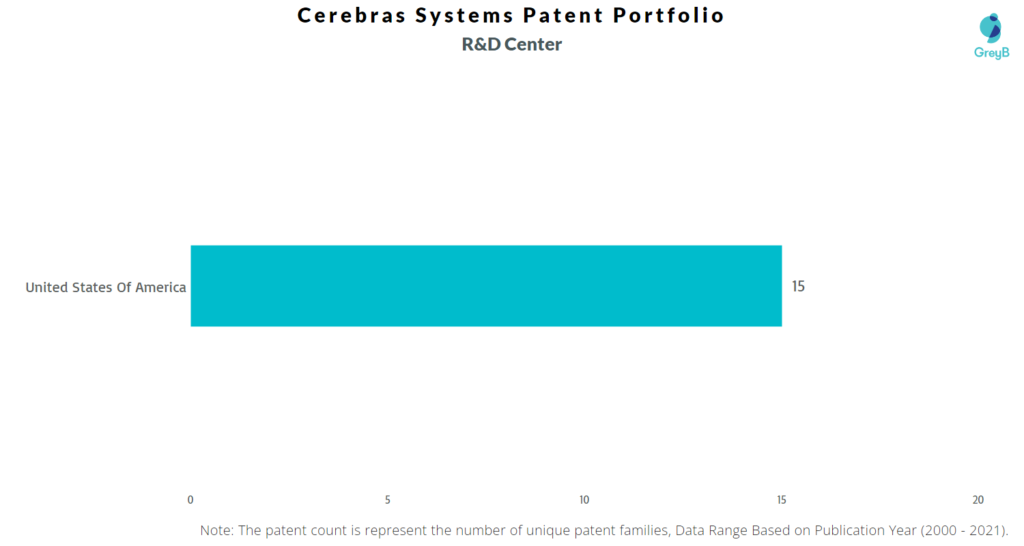
10 Best Cerebras Systems Patents
US20200005142A1 is the most popular patent in the Cerebras Systems portfolio. It has received 8 citations so far from companies like Sony Corp, Intel Corporation and International Business Machines Corporation.
Publication Number | Citation Count |
US20200005142A1 | 8 |
WO2018193377A1 | 8 |
WO2018193360A1 | 8 |
WO2018193354A1 | 8 |
US10515303B2 | 7 |
WO2020021395A1 | 7 |
WO2018193380A1 | 7 |
WO2018193379A1 | 7 |
WO2018193370A1 | 7 |
WO2018193363A1 | 7 |
What Percentage of Cerebras Systems US Patent Applications were Granted?
Cerebras Systems (Excluding its subsidiaries) has filed 37 patent applications at USPTO so far (Excluding Design and PCT applications). Out of these 22 have been granted leading to a grant rate of 100%.
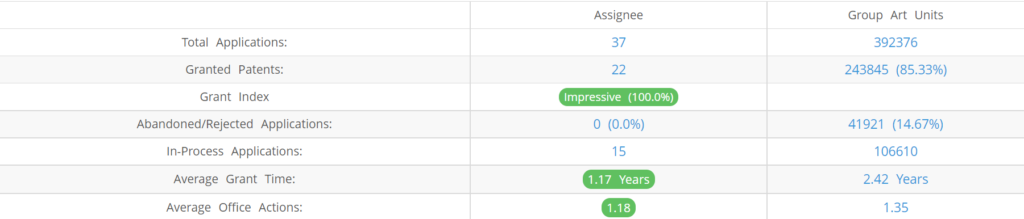
Which Law Firms Filed Most US Patents for Cerebras Systems?
Law Firm | Total Application | Success Rate |
Schox Pc | 24 | 100% |
Patentventures Cs | 13 | 100% |
“We have come together to build a new class of computer to accelerate artificial intelligence work by three orders of magnitude beyond the current state of the art.”
Cerebras Systems is the pioneer in high performance artificial intelligence computing and Peptilogics. It has been named to Fast Company’s prestigious annual list of the World’s Most Innovative Companies for 2021.
What are Cerebras Systems’s key innovation segments? What can you expect next??
List of Cerebras Systems Patents
Publication Number | Title (English) |
EP3607506B1 | Fabric vectors for deep learning acceleration |
US11062202B2 | Numerical representation for neural networks |
US11062200B2 | Task synchronization for accelerated deep learning |
CN110869946B | Accelerated deep learning |
JP06860694B2 | The task activation of acceleration deep learning |
JP06854473B2 | The data-flow trigger task of acceleration deep learning |
US10971401B2 | Systems and methods for precision fabrication of an orifice within an integrated circuit |
US10957595B2 | Systems and methods for precision fabrication of an orifice within an integrated circuit |
CA3060356C | Task activating for accelerated deep learning |
CA3051990C | Accelerated deep learning |
JP06832050B2 | Acceleration deep learning |
US10923456B2 | Systems and methods for hierarchical exposure of an integrated circuit having multiple interconnected die |
US10923412B2 | Apparatuses and methods for implementing a sliding thermal interface between substrates with varying coefficients of thermal expansion |
US10892244B2 | Apparatus and method for securing substrates with varying coefficients of thermal expansion |
CA3060969C | Neuron smearing for accelerated deep learning |
US10840216B2 | Systems and methods for powering an integrated circuit having multiple interconnected die |
US10784128B2 | Apparatus and method for securing components of an integrated circuit |
JP06755541B2 | Neuron smearing of acceleration deep learning |
US10777532B2 | Apparatus and method for multi-die interconnection |
US10762418B2 | Control wavelet for accelerated deep learning |
US10726329B2 | Data structure descriptors for deep learning acceleration |
CA3060368C | Dataflow triggered tasks for accelerated deep learning |
US10699189B2 | Accelerated deep learning |
US10672732B2 | Apparatus and method for securing substrates with varying coefficients of thermal expansion |
US10657438B2 | Backpressure for accelerated deep learning |
US10614357B2 | Dataflow triggered tasks for accelerated deep learning |
US10586784B2 | Apparatus and method for multi-die interconnection |
US10515303B2 | Wavelet representation for accelerated deep learning |
US10468369B2 | Apparatus and method for securing substrates with varying coefficients of thermal expansion |
US10453717B2 | Apparatus and method for securing components of an integrated circuit |
US10366967B2 | Apparatus and method for multi-die interconnection |
US10361172B2 | Apparatus and method for multi-die interconnection |
US10332860B2 | Apparatus and method for multi-die interconnection |
US10242891B2 | Apparatus and method for securing components of an integrated circuit |
US20210256362A1 | Processor element redundancy for accelerated deep learning |
US20210255860A1 | Isa enhancements for accelerated deep learning |
US20210248453A1 | Scaled compute fabric for accelerated deep learning |
JP2021108157A | Task activating for accelerated deep learning |
JP2021108131A | Neuron smearing for accelerated deep learning |
US20210224639A1 | Control wavelet for accelerated deep learning |
US20210167037A1 | Systems and methods for hierarchical exposure of an integrated circuit having multiple interconnected die |
US20210166109A1 | Data structure descriptors for deep learning acceleration |
JP2021082317A | Acceleration deep learning |
EP3659178A4 | Apparatus and method for securing substrates with varying coefficients of thermal expansion |
US20210143041A1 | System and method for alignment of an integrated circuit |
US20210142167A1 | Accelerated deep learning |
US20210134700A1 | Apparatuses and methods for implementing a sliding thermal interface between substrates with varying coefficients of thermal expansion |
WO2021084506A1 | Distributed placement of linear operators for accelerated deep learning |
WO2021084505A1 | Optimized placement for efficiency for accelerated deep learning |
WO2021084485A1 | Placement of compute and memory for accelerated deep learning |
US20210125871A1 | Systems and methods for precision fabrication of an orifice within an integrated circuit |
EP3659055A4 | Apparatus and method for multi-die interconnection |
WO2021074867A1 | Advanced wavelet filtering for accelerated deep learning |
WO2021074865A1 | Basic wavelet filtering for accelerated deep learning |
WO2021074795A1 | Dynamic routing for accelerated deep learning |
US20210097376A1 | Backpressure for Accelerated Deep Learning |
US20210091035A1 | Apparatus and method for securing substrates with varying coefficients of thermal expansion |
EP3610424A4 | Data structure descriptors for deep learning acceleration |
US20210056400A1 | Dataflow Triggered Tasks for Accelerated Deep Learning |
KR2021014056A | Accelerated deep learning |
US20210004674A1 | Task activating for accelerated deep learning |
US20200402957A1 | Systems and methods for powering an integrated circuit having multiple interconnected die |
JP2020205067A | Neuron smearing for accelerated Deep Learning | Neuron smearing of acceleration deep learning |
US20200381394A1 | Apparatus and method for multi-die interconnection |
US20200381274A1 | Apparatus and method for securing components of an integrated circuit |
US20200380370A1 | Floating-point unit stochastic rounding for accelerated deep learning |
US20200380344A1 | Neuron smearing for accelerated deep learning |
US20200380341A1 | Fabric Vectors for Deep Learning Acceleration |
US20200364546A1 | Wavelet representation for accelerated deep learning |
EP3610612A4 | Dataflow triggered tasks for accelerated deep learning |
HK40016392A | Accelerated deep learning |
JP2020517030A | Neuron smearing for accelerated deep learning |
CN111095527A | Device and method for multi-die interconnection |
EP3607505A4 | Task synchronization for accelerated deep learning |
EP3607503A4 | Task activating for accelerated deep learning |
US20200125934A1 | Microthreading for accelerated deep learning |
EP3607504A4 | Neuron smearing for accelerated deep learning |
WO2020044238A1 | Processor element redundancy for accelerated deep learning |
WO2020044208A1 | Isa enhancements for accelerated deep learning |
WO2020044152A1 | Scaled compute fabric for accelerated deep learning |
WO2020021395A1 | Numerical representation for neural networks |
EP3563307A4 | Accelerated deep learning |
US20200005142A1 | Accelerated deep learning |
WO2019040273A1 | Apparatus and method for securing components of an integrated circuit |
WO2019022942A1 | Apparatus and method for securing substrates with varying coefficients of thermal expansion |
WO2019022902A1 | Apparatus and method for multi-die interconnection |
WO2018193380A1 | Fabric vectors for deep learning acceleration |
WO2018193379A1 | Backpressure for accelerated deep learning |
WO2018193377A1 | Control wavelet for accelerated deep learning |
WO2018193370A1 | Task activating for accelerated deep learning |
WO2018193363A1 | Data structure descriptors for deep learning acceleration |
WO2018193361A1 | Microthreading for accelerated deep learning |
WO2018193360A1 | Task synchronization for accelerated deep learning |
WO2018193354A1 | Wavelet representation for accelerated deep learning |
WO2018193353A1 | Neuron smearing for accelerated deep learning |
WO2018193352A1 | Dataflow triggered tasks for accelerated deep learning |
CA3108089A1 | Task activating for accelerated deep learning |
CA3099965A1 | Neuron smearing for accelerated deep learning |
CA3060350A1 | Data structure descriptors for deep learning acceleration |
WO2018189728A1 | Floating-point unit stochastic rounding for accelerated deep learning |
WO2018154494A1 | Accelerated deep learning |
CA3108151A1 | Accelerated deep learning |