In recent scientific breakthroughs, one innovation stands tall: Alphafold by Google DeepMind. This AI-driven platform is transforming the field of biology, predicting protein structures with remarkable precision. With the introduction of Alphafold 3 and its powerful Alphafold multimer capabilities, researchers are unlocking new potential in drug discovery, medical research, and biotechnology.
But what exactly makes Alphafold such a game-changer, and why are scientists worldwide hailing it as the future of protein research?
Whether you’re a researcher looking for faster protein complex solutions or simply curious about the technology behind it, Alphafold’s story is one of AI at its best. Let’s explore the key patents of this cutting-edge platform and what sets it apart.
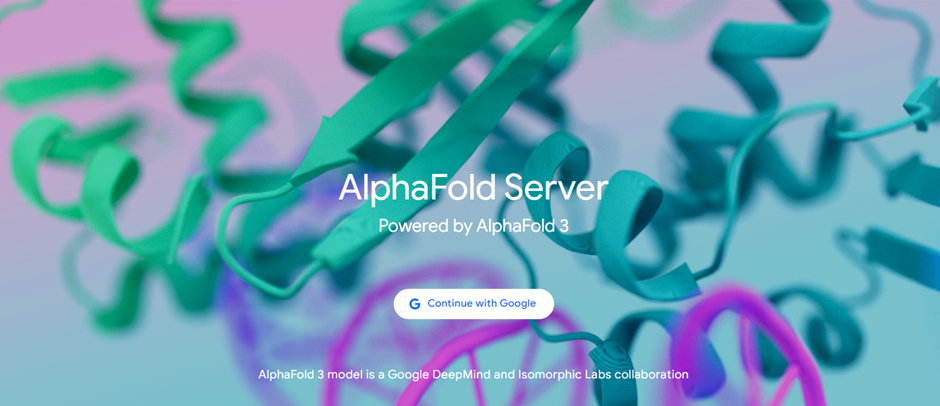
How Does Alphafold Work?
Alphafold is more than just an AI model—it’s a breakthrough in computational biology. Using deep learning algorithms, Alphafold predicts the 3D structure of proteins based on their amino acid sequences.
The system takes advantage of data from tens of thousands of protein structures, learning to refine predictions with each iteration. Its groundbreaking Alphafold ab initio capability allows it to predict protein structures from scratch without relying on prior templates. This opens up vast possibilities in medical research and drug discovery, making it an indispensable tool for scientists around the globe.
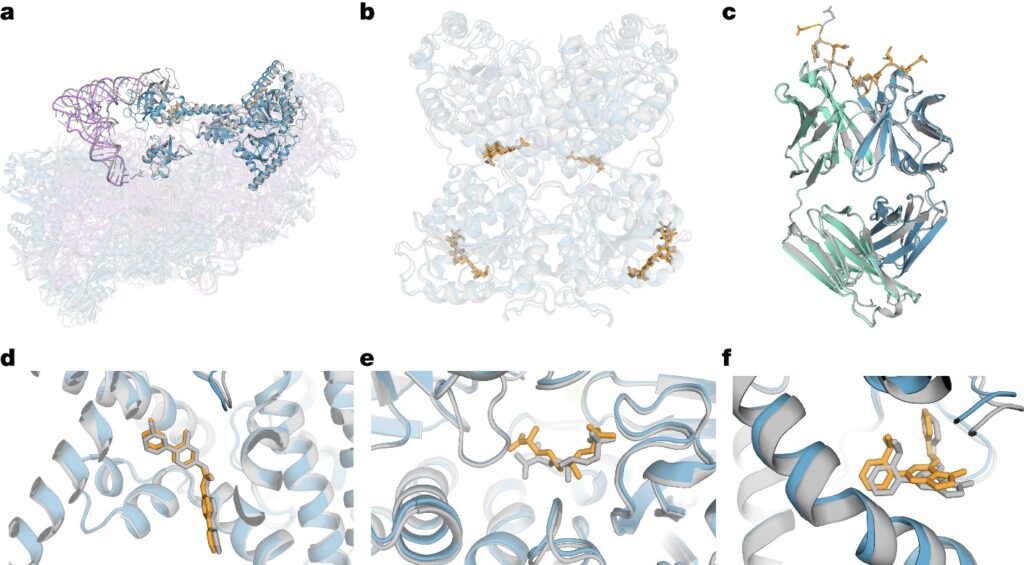
What Makes Alphafold So Unique?
At the heart of Alphafold’s success lies its innovative use of artificial intelligence and deep learning. Here’s what makes Alphafold stand out:
- AI-Driven Protein Prediction: By employing machine learning, Alphafold iteratively improves its predictions of protein structures, refining bond angles and distances to increase accuracy.
- Distance Mapping: Alphafold uses distance maps to enhance the structural predictions, a feature that gives it an edge in accuracy over traditional methods.
- Multi-Chain Protein Predictions: With its Alphafold multimer function, Alphafold can now predict the structures of complex protein assemblies, allowing researchers to study protein interactions like never before.
- 3D Modeling from Cryo-EM: Alphafold leverages cryo-EM images to produce 3D models of proteins, giving scientists a clear view of proteins in various conformations, an essential step for understanding how proteins behave in different environments.
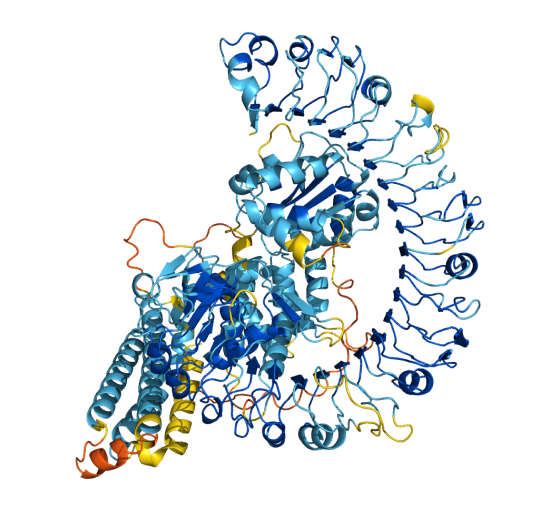
What Key Patents Drive Alphafold’s Innovation?
The secret behind Alphafold’s unmatched accuracy lies in the patents that protect its cutting-edge methods:
- JP7132430B2 (Machine Learning for Determining Protein Structures)
This patent covers Alphafold’s use of neural networks for refining protein structures iteratively, ensuring more precise predictions.
- US20220415453A1 (Determining Atom Coordinates of Macromolecules from Images Using Auto-Encoders)
This patent focuses on generating 3D structures of proteins using cryo-EM images, contributing to Alphafold’s high accuracy in modeling protein conformations.
- US20210398606A1 (Protein Structure Prediction Using Geometric Attention Neural Networks)
Alphafold’s neural network folds proteins by adjusting the spatial locations and rotations of amino acids, a method detailed in this patent that drives Alphafold’s success.
- WO2023057455A1 (Predicting Multi-Chain Protein Structures)
The capability to predict the structure of multimeric proteins—complexes involving multiple protein chains—comes from this patent, setting Alphafold apart from its competitors.
Check out the list below for the key patents related Google DeepMind’s Alphafold:
Patent Number | Patent Title |
JP7132430B2 | Machine Learning For Determining Protein Structures |
US20220415453A1 | Determining A Distribution Of Atom Coordinates Of A Macromolecule From Images Using Auto-Encoders |
US20220172055A1 | Predicting Biological Functions Of Proteins Using Dilated Convolutional Neural Networks |
US20210398606A1 | Protein Structure Prediction Using Geometric Attention Neural Networks |
US20210166779A1 | Protein Structure Prediction From Amino Acid Sequences Using Self-Attention Neural Networks |
US20190295688A1 | Processing Biological Sequences Using Neural Networks |
WO2023057455A1 | Training A Neural Network To Predict Multi-Chain Protein Structures |
WO2022194434A1 | Predicting Complete Protein Representations From Masked Protein Representations |
WO2022167325A1 | Predicting Protein Amino Acid Sequences Using Generative Models Conditioned On Protein Structure Embeddings |
WO2022112220A1 | Predicting Symmetrical Protein Structures Using Symmetrical Expansion Transformations |
WO2022112248A1 | Predicting Protein Structures By Sharing Information Between Multiple Sequence Alignments And Pair Embeddings |
WO2022112255A1 | Predicting Protein Structures Using Protein Graphs |
WO2022112257A1 | Predicting Protein Structures Using Auxiliary Folding Networks |
WO2022112260A1 | Predicting Protein Structures Over Multiple Iterations Using Recycling |
WO2022089805A1 | Training Protein Structure Prediction Neural Networks Using Reduced Multiple Sequence Alignments |
Who Competes with Alphafold?
While Alphafold dominates the protein prediction landscape, it isn’t without competition. Here are some notable competitors and how Alphafold compares:
- Swiss Model: Swiss Model provides template-based predictions, but its dependency on existing protein structures limits its flexibility, especially when it comes to modeling novel proteins or protein complexes compared to Alphafold’s AI-driven predictions, especially for multimeric proteins.
- RoseTTAFold: This tool uses a similar deep learning approach but lacks the comprehensive accuracy and speed of Alphafold, particularly when it comes to handling protein complexes.
- I-TASSER: A long-standing tool in protein prediction, I-TASSER relies on template models, which can hinder its accuracy when there are no pre-existing structures to reference, unlike Alphafold’s ab initio approach.
Why Should Researchers Choose Alphafold?
Alphafold is more than just another protein structure prediction tool—it’s leading a paradigm shift in biological research. Here’s why researchers are increasingly choosing Alphafold:
- AI Precision: Alphafold’s AI-powered models ensure precise predictions, even for complex protein structures, making it an invaluable tool for research.
- Complex Protein Predictions: With Alphafold multimer, Alphafold is unrivaled when it comes to predicting the structures of multimeric proteins, which are essential for studying protein-protein interactions.
- Speed and Efficiency: Alphafold’s efficiency and user-friendly Alphafold 3 server allow researchers to obtain results quickly, offering faster insights than competing tools.
As the world of biotechnology advances, Alphafold is leading the charge in protein structure prediction. Its combination of speed, precision, and versatility makes it an essential tool for researchers and a beacon of what artificial intelligence can achieve in science. Whether it’s protein complex prediction or handling multimeric proteins, Alphafold is shaping the future of protein research.
What other companies are filing patents in the AI-driven protein folding technology? Request a patent landscape around this technology by filling out the form below: